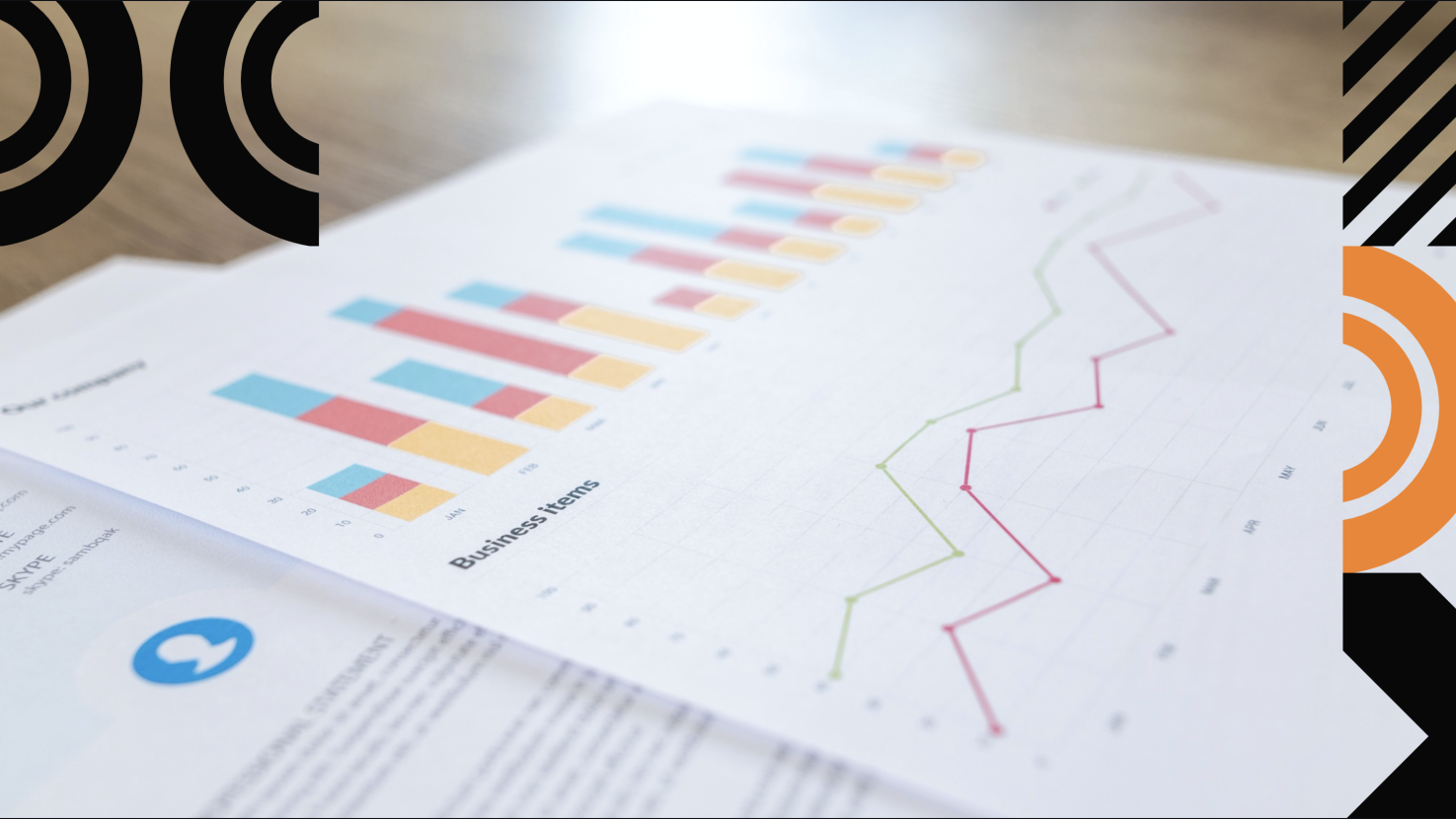

The Value of Data Governance: Where’s the ROI?
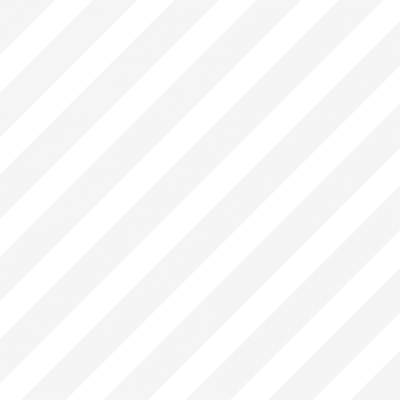
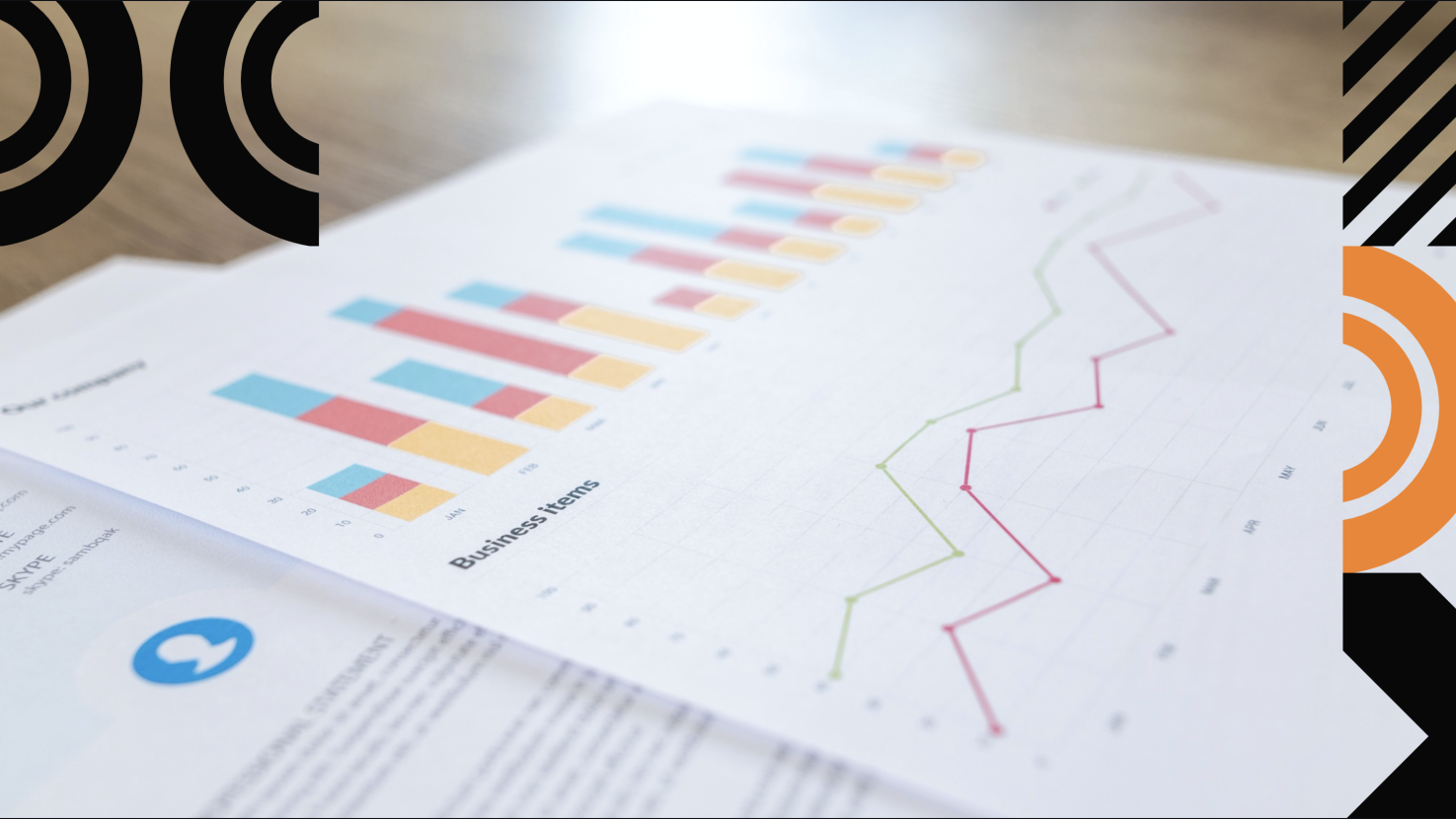
If it didn’t happen, can you claim ROI on it?
That’s part of the conundrum with identifying value from a data governance program. Governance is typically associated with reducing risk, but it’s impossible to measure what didn’t happen.
Beyond just measuring data governance ROI, another challenge is that most data leaders are grappling with a demand for their services that far exceeds their ability to meet it. This adds additional pressure to justify what they’re doing, which makes data governance an awkward thing to own if you’re measured on returns alone.
Against this backdrop, organizations are moving towards agile as our modus operandi. Part of the reason is a belief that we have no idea what the world will look like in four years time, so why would we set up waterfall product development and release cycles with massive timelines?
That raises hard questions for data governance where long timelines seem to be par for the course. I once had a vendor tell me not to expect any value from a data catalog product I was considering for three to four years. Needless to say, their company didn’t make my short list. Their path to demonstrable value of data governance was far longer than the average tenure of the CDO and certainly longer than the timelines I was measured on or believed I could deliver to.
Govern people and their activities
Here’s one way to think about a solution.
An irony I often come across in data governance is the focus on data. Yes, data streams in real time and there is always more of it, but data is inert. It doesn’t do anything of its own accord. Therefore it’s a core misjudgment in my view to think you can “govern” data. You can only govern the activities around data.
To truly see data governance’s value, companies should be weaving governance directly into their existing data activities rather than seeing it as a separate activity. For example, you should work to discover how your central data engineering team manages its work, look at that process, and understand points where you might be able to improve data governance, preferably without slowing those teams down.
Or if you’re a large organization starting its cloud initiative, you’re going to have a program going on to ingest data for several quarters. If you added data governance controls to the work that the ingest team did, I believe you’d achieve many of the aims of data governance just as part of that initiative.
The dialog could run something like this:
“Cloud ingestion team, what is it you are trying to achieve?”
“We believe we need to achieve replicating the data from X number of systems and legacy data marts onto the cloud.”
“Perfect. The organization is also struggling with data searchability and access issues. Could we classify the data and enable default access to proprietary, non-personal data at the same time?”
I think this method of governance, which is more around governing activity than data, makes governance a lot easier. For example, a common problem when approaching governance from the data backwards is struggling to find data owners for data sets. Many candidates to be a data owner say they lack the technical teams to manage the data and help them fulfill their role as owner. If you approach governance from the activities backwards every data set is already being worked on by technical teams, so it is easy to find data owners. The owner is the accountable person for the team working on the data, at least until a more appropriate owner can be found.
Is governance the right term?
Some have noted that governance might be a bit haughty as a term, but I think it works to describe this kind of management activity. It tends to give senior sponsors a lot of comfort. And every consultancy I’ve ever seen that’s done a data assessment likes to talk about things relative to governance. To me changing what it means in execution is easier than rebranding it.
On the other hand, it is interesting to ask if data engineering should own data governance? I believe they should certainly have a very large say in it. The data engineering team are likely doing most of the complex data work and they define many technical standards for how data is used within an organization. And in my experience data engineering teams are constantly evolving and redefining those technical standards.
If someone outside of that very fast-moving system is setting rules without really understanding what’s going on in that environment, it sounds to me like a lot of added friction and much higher odds for miscommunication. My solution would be to merge data governance and engineering teams and to make all heads of data engineering responsible for data governance in their areas.
Where data leaders should step up
How can data leaders better collaborate with other parts of the organization around data governance, if that collaboration is not going on right now?
One point I believe in very strongly is that in large organizations it is impossible to centrally lead all data strategy and large organizations should eventually move to a multiple CDO model. That’s because data activities and regulations vary quite a lot by different domains. Central data strategy and governance is still essential for collaboration and avoiding data silos. But collaboration gets easier when spokes in the functions can take accountability for their domain specific challenges. For example, finance considering the implication of Sarbanes-Oxley for data management or customer/commercial function owning the brand aspect of customer data management. Typically this requires data ownership at the director level in the functions and a multiple CDO model.
I hold this position because the challenge with data governance when you come into an immature organization is that forums with the authority to drive change require senior attendees. But senior attendees often don’t have a clue about how people work with data at a practical level or have the time to listen to practical data challenges from domains that are not relevant to them.
This makes it very hard to collaborate, because the central data team – which might have a director and a senior director – are working with managers who can’t implement different ways of doing things. Yet if they were to work with someone at a more senior level, it’s less likely that they’ll actually understand the topic. That’s why bringing data maturity up a few notches is key. Eventually those functions do need more senior roles with a technical bent to them. What you want is staff with the ability to collaborate with someone who understands some of the technical nuance you need to have these conversations but also connects to stakeholders’ sense of the functional priorities and how to drive change in their area.
That’s when you can truly collaborate and see impact. Instead of senior-level people attending meetings out of politeness while not understanding the topics seated next to junior-level people who know exactly what’s going on but have no remit to change anything, we should aim to build higher data maturity among people who do have organizational authority. In the case of data governance, this can mean understanding enough about the true role of data engineering to collaborate with them on it and share the controls.
And doesn’t it sound a lot more effective and enjoyable to govern together rather than apart?