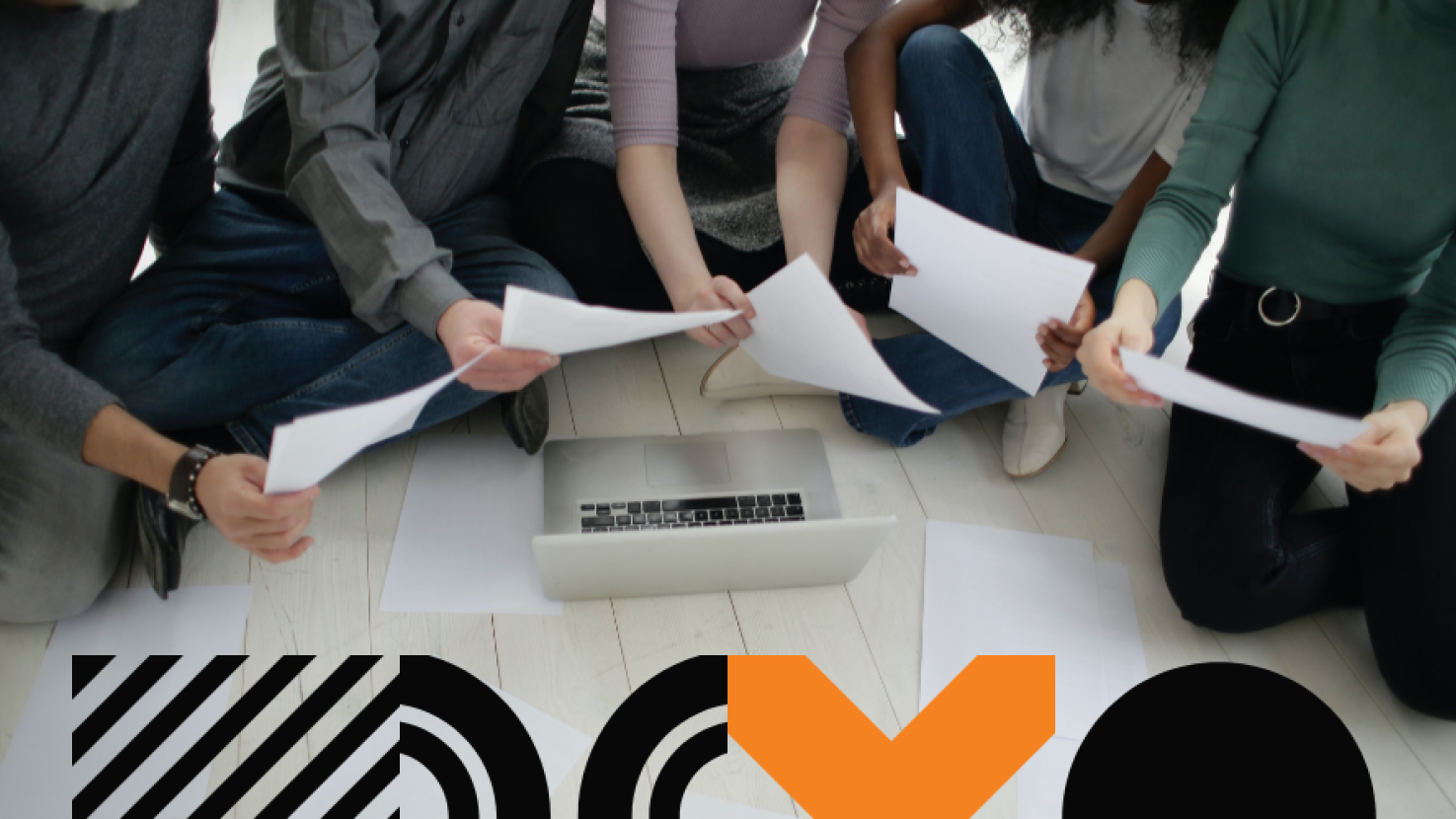

6 Strategies to Assess and Advance Data Governance Maturity
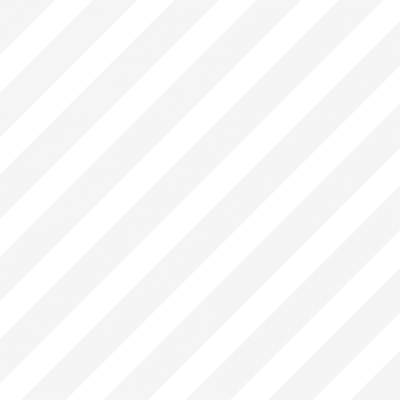
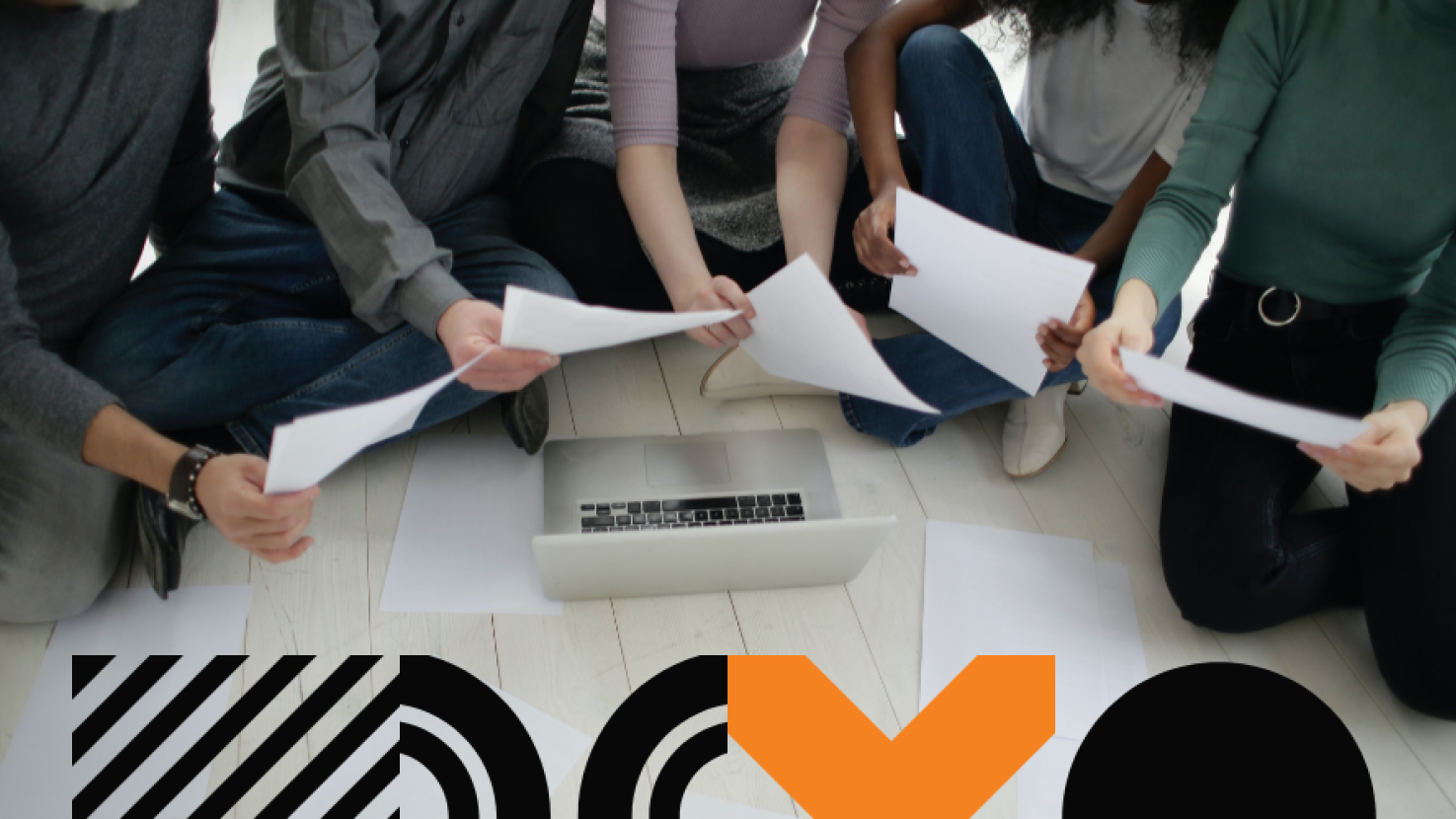
Before you can improve upon your current data governance practices, you must first measure where you are today. But what is it you’re measuring? Here are a few misconceptions that I continue to see in my career when it comes to data governance:
- Data governance is only about compliance. Compliance does play a vital role in governance, but it is much more than a bunch of policies that need to be adhered to. Data quality is a huge part of data governance, and poses many real-world questions that have an impact on our daily work: Is my data accurate? Is it complete? Is data available when it’s needed it? Accessibility is part of governance, too. Who has access to certain data assets, and under what circumstances? It’s a much larger umbrella than people may realize.
I like to think of governance in a way that anyone in the business can understand: it covers everything that has to do with ensuring that the data of your organization continues to remain a valuable asset.
- It should sit within IT. People who don’t know governance well assume by default that it’s an IT function. In reality, it should be a business function. That’s because the goal of data governance as a concept is to support and drive business objectives. This is why data leaders should ensure their governance strategies are aligned with business goals. This enables better collaboration and engagement with various stakeholders across the business given that data is versatile and used differently based on specific needs.
- It has a start and end date. If data was static, this might be true, but data governance is an ongoing initiative that continues to evolve. This evolution is driven by changing business requirements, changes in the business environment, as well as technology advancements. The advent of ChatGPT is a perfect example. As advanced natural language processing tools like ChatGPT become increasingly central to business activities, the questions surrounding governance also evolve. For instance, how do we govern data that is consumed by AI applications? and what does this mean for our governance policies?
Why assess your data governance maturity
Precisely because data governance is a continuous initiative, every organization that runs on data needs to pause periodically to evaluate and see where they are in the data journey.
Take for example an e-commerce company that is trying to leverage predictive analytics to focus the demand of its customers and optimize its supply chain. To pursue these initiatives, they obviously would be leveraging data. This is exactly when a data leader should scrutinize data records for inconsistencies in customer data, sales data, records, purchases, and data records. Are there data inconsistencies, duplicates, and errors? Any such issues could significantly affect the outcomes achieved by their analytics solution and should be identified during a data governance maturity assessment.
Part of the recommendations of the assessment result here could be to establish a data governance stewardship of responsibility and then probably assign roles to who needs to do what. For example, who needs to track and measure data quality, who needs to track data quality metrics, and how are data quality issues to be remediated? Without a data governance maturity assessment, many of these issues might not surface until the company had spent a lot of time and money on a solution that would not deliver much in the way of results.
Committee, model, measurement, framework
Who do you tap to complete the data governance assessment as a data leader? Ideally, you would turn to one person who can see and explain all the issues from a business and an IT perspective. But these data unicorns are rare because of the way our education system is structured. You either are a developer studying code or you are the businessperson. In between these two domains, organizations need to speak to each other, so choosing the right committee of technical and business-minded people is vital, as is keeping them focused on implementation and execution as well as definition of the data governance program.
There are a lot of models that can be used to assess data governance maturity as well. Smaller organizations must be cautious not to adopt a model used by larger organizations because the experience is likely to be overwhelming. Here it makes sense to select a model that can be flexible and will easily align to the expectations of the assessment.
Key performance indicators are the third part of a successful assessment. Data quality will almost always be a priority here because it is a precursor to so many other objectives and indicators. Depending on resources, though, it may not be realistic to achieve significantly higher data quality across all domains. In the beginning, it may be that you ensure documentation of data lineage of critical data elements for preparation of a group of key reports. For example, a data maturity indicator might be 80% data quality in these reports’ tables in terms of completeness, accuracy, and little to no duplications, with associated controls around each of these processes.
Finally, of course, there is establishing a data governance framework. A key result indicator here would be to ensure that we have policies and procedures documented across functions and departments in the organization. This becomes a measurable outcome that one can actually use as a benchmark when the data quality governance and maturity assessment is done.
Proving the value
Any data leader who has been involved in data governance knows that it can be very challenging to come up with the value that data governance is actually bringing to the table. That’s because governance usually involves investment upfront and takes a lot of work to convince senior leaders that this will be valuable to the organization if you do it. That’s one reason why most data governance initiative are triggered by regulatory requirements.
Of course, a skilled data leader can go much further than arguing that regulations say we must assess and build our data governance framework. Six strategies I’ve seen to be successful are:
- Translate technical data into business terms. High level executives typically aren’t concerned with specifics of applications or technology infrastructure required for effective data governance. That’s why it's important to speak their language. Making an effort to translate the data governance initiative in terms of financial impact makes this task very easy.
- Dramatize the price of inaction. It’s also effective to cite examples of organizations or companies that have failed or risked failure by not adopting good data governance. A lot of organizations have been fined because of data issues. Senior leaders are typically very good at relating to this risk
- Start with a pilot. A pilot project in data governance shouldn’t require an enormous budget or an extended timeline. Executing a successful pilot can build trust, showcase tangible results, and provide a clearer picture of the broader requirements.
- Engage champions. There are influential stakeholders in any organization. They can advocate to senior executives with you or on your behalf. Identifying them, collaborating with them, and having them support your data governance project will help to ensure its success
- Share observations and set priorities. Every data governance assessment will produce observations in terms of what you’re doing well and what you need to improve upon to get onto that next level of data maturity. Rather than keeping these findings within the data team, it’s more productive to discuss them with your business peers and ask, “Given these findings, what do you think is of priority to you, in terms of the business?” For example, if your business goal is a 360-degree view of the customer but the assessment says that all data is actually maintained in silos, you and your business leadership need to discuss an investment in a person or an application that will help support data integration.
- Create an action plan. Once you decide on your business priorities, you need an action plan. As the saying goes, if we fail to plan, we plan to fail. This works by breaking the plan into different milestones and identifying who is responsible for what. You also need to check for resources, funds, and the necessary skills. Finally, there needs to be a discussion with the key stakeholders that will be impacted by the plan: how much time they’ll need to contribute, when check-ins occur, how escalations will work. They also need to understand the value that the initiative is going to bring them. Otherwise, there’s going to be a lot of pushback when the projects start.
Embrace the complexity
Yes, data governance can be a complex process. It’s ongoing and continuous. However, the value it adds to your organization is undeniable. Ascending along the maturity curve will bring many benefits such as higher efficiency, more successful initiatives that rely on quality data, and competitive advantage among your peers. These are benefits everyone in an organization can embrace.