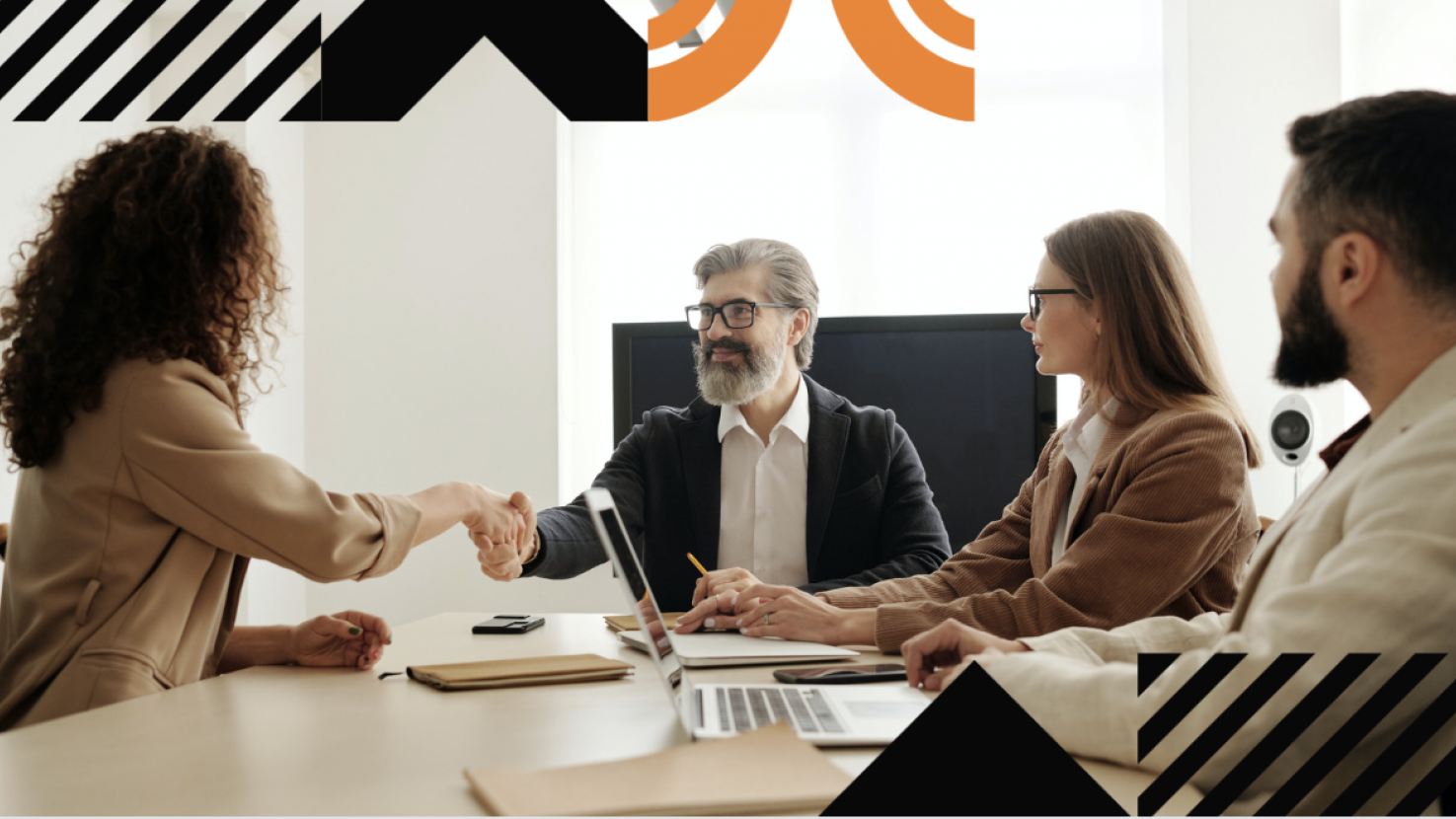

Where Governance Meets Stewardship: Getting to Trusted Data Faster
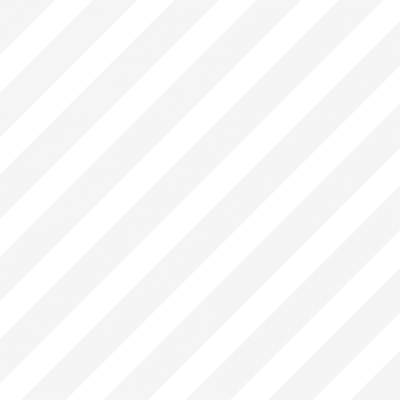
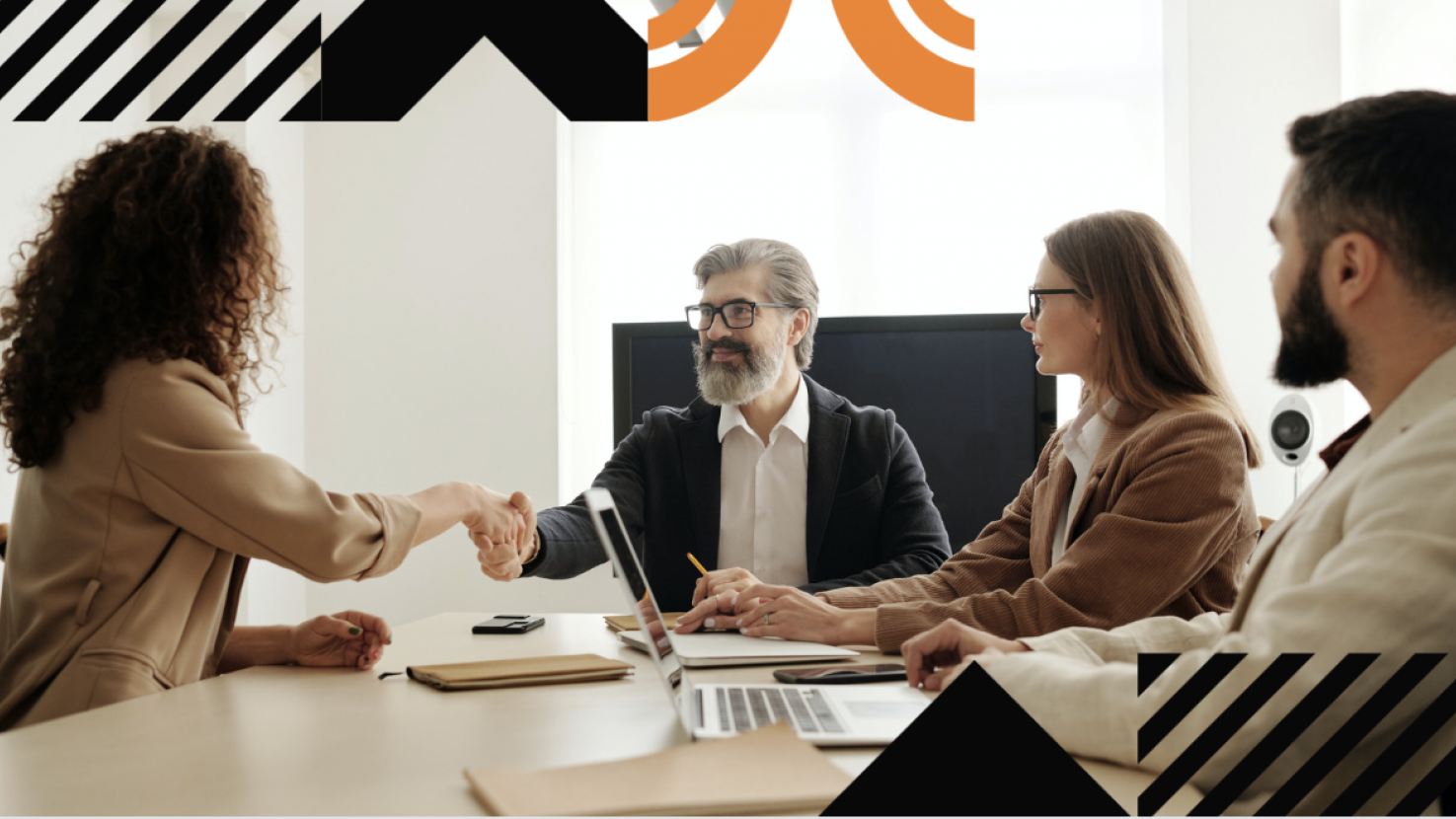
Do you trust your data?
Posing this question addresses one of the most important, but least represented or appreciated, aspects of data analytics. Because they’re pressed for time and being looked to for their judgments, senior executives often default to saying, “Just tell me what’s going to happen” without appreciating the single question about data trust that everything they’re seeing is built upon.
I consider myself fortunate to have worked with a manager who said, “We’ve got to care about this stuff.” Because of his mentorship, data quality became one of my most important priorities.
Governance + Stewardship
Every data leader will have their own formula for data quality, but I follow a combination of governance and enablement (or what I call stewardship) by the employees.
- governance, because there must be a central team to oversee data quality
- stewardship, because we all own data quality at the end of the day
The data governance team monitors data quality health, which should include data trust solutions like programs to score data and ensure that confidence in it extends from the analytics teams to users.
Beyond data governance, I believe data leaders also should consider what I call a data stewardship team. This larger group of trusted users has a very specific charter: as soon as they come across data that fails to meet standards in some way, they report in on that variance. If something is out of variance for a specific reason, the data governance team could adjust that centrally rather than on a piecemeal or ad hoc basis.
Of course, not every variation in data needs to be elevated to the data governance team. That’s why it’s wise to follow a bimodal model: employees should be able to address and correct simple data mistakes themselves, or easily push the observed variance up the chain to the governance team if they don’t feel comfortable doing it. Simply saying, “We’re all in charge of data quality” doesn't mean anything if you don't have a path to fix it, whether that’s a marketing database or CRM system. Enabling an “alarm button” when the scope is too great for one person ensures that you’re only a click away from knowing there may be a problem.
Staying accountable
Once this system is up and running, it’s important to conduct ongoing audits to ensure that the company is accountable to its own data. Another successful strategy is to centralize definitions of critical metrics in the data set itself, and document it appropriately, so that everyone isn’t trying to calculate a critical metric in different ways. Creating and distributing key metrics as part of your data strategy is a highly effective way to ensure everyone stays aligned.
As I see it, even the most trusted data is just data until it’s put to good use.