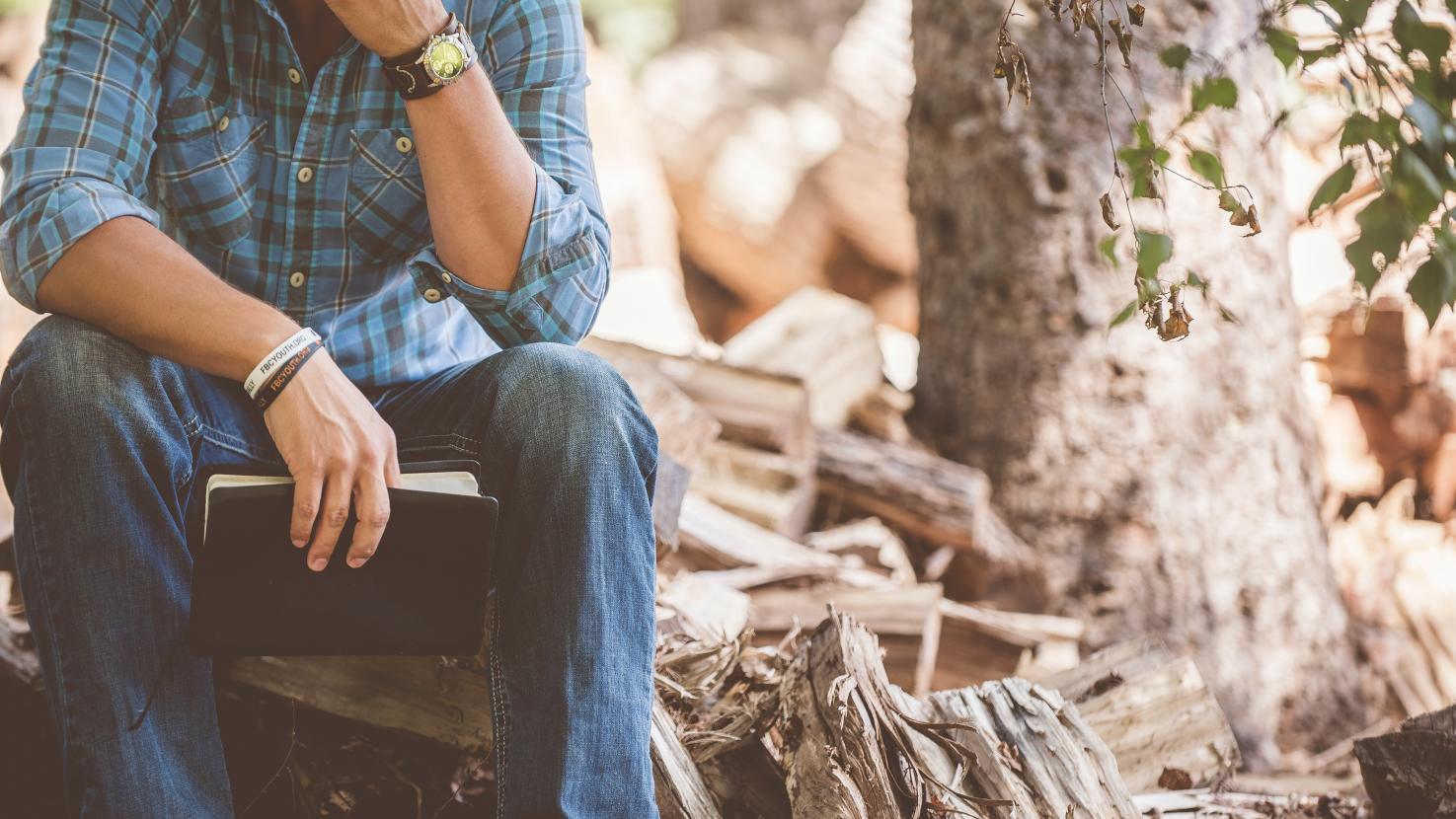

Three Data Management Questions You Need to Ask
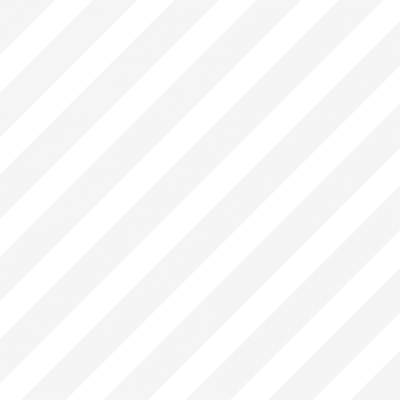
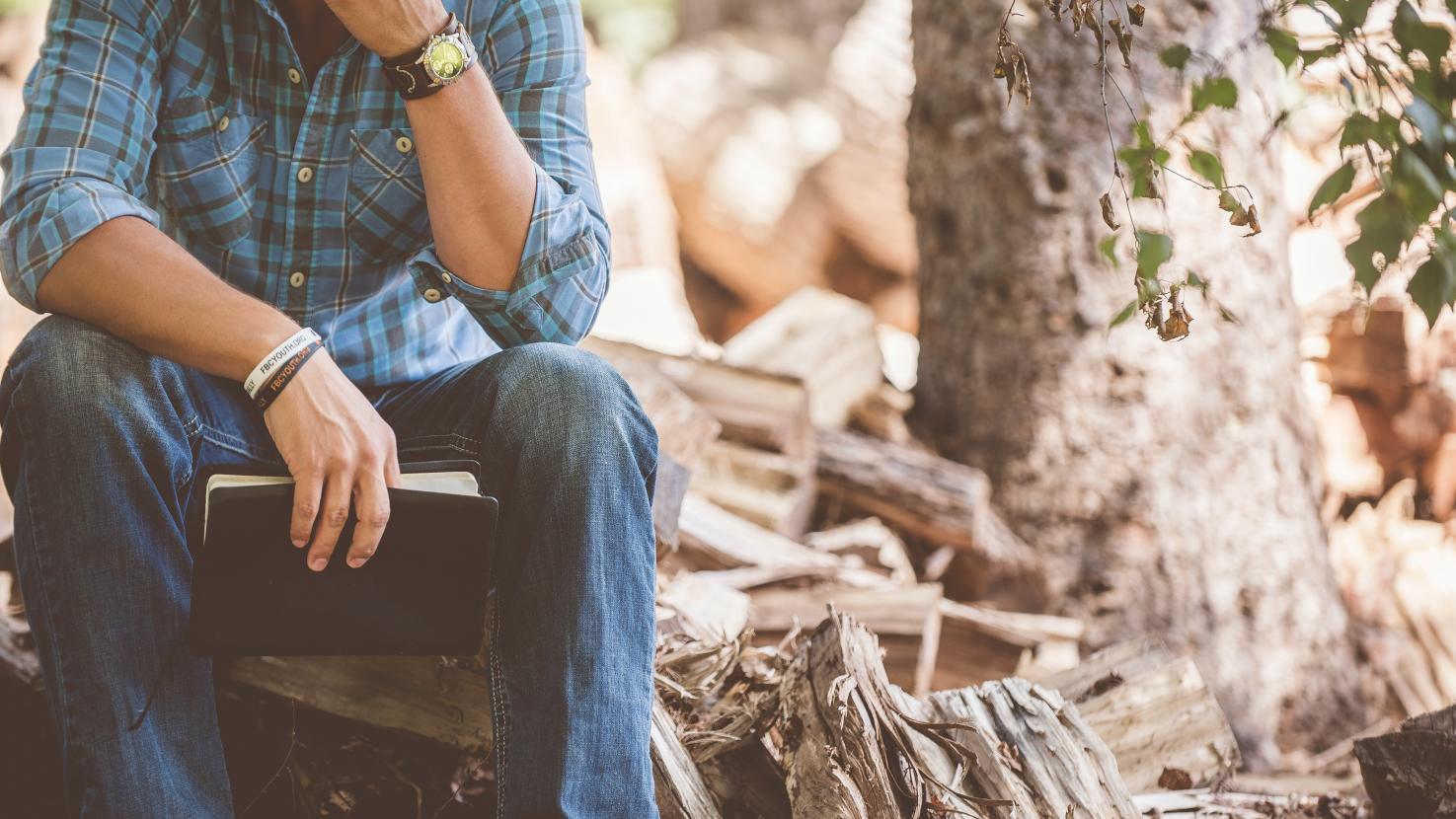
Having a business outcomes-centric perspective is critical to success these days, because so many companies are attempting to move their data management infrastructure to the cloud and take advantage of machine learning and AI capabilities that can have a transformative impact on their data and analytics performance.
Before they reach their desired state, though, data leaders are typically confronted by three questions:
- Where does the data that we need currently live?
- Can we get our data to where it actually needs to go?
- Do we have data quality issues that undermine user trust?
All three of these data management questions represent points where companies typically slow down or get stuck, so each needs to be considered carefully in terms of pertinent solutions. Let’s walk through each of these data management questions and their answers.
Question 1: Where Is My Data?
Many enterprises have a crazy, complex data landscape, often defined by technology debt and legacy solutions spanning on-premises and multiple cloud environments, that makes it difficult to discover the data assets they need to consider. Data leaders rightfully ask themselves: “Can we get to a technical view of what we have? Do we have dark pockets of data hiding out there that we’re not aware of?”
Solution: Data Catalogs
Any company trying to get their arms around their data needs a tool like a data catalog that can go out into your environment, scan all your existing cloud and on-prem data sources, and use machine learning to classify and organize it in terms of what it means to the business. Once your data is scanned and categorized, it becomes far easier to ask, for example, "Okay, where’s my customer data?" without having to know the name of the tables or columns where it resides.
Question 2: Can we get our data out of where it lives to where it needs to go?
Answering this question tends to be a connectivity and data transformation/preparation problem.
Solution: Cloud Data Integration
Moving, cleaning, deduplicating, combining and placing structured and unstructured data into the right database or data warehouse is commonly known as data integration. Here, too, the set of tools, the data they handle, and where the processing happens has evolved rapidly as these tools have moved to the cloud. In the cloud, you can scale data integration up and down and allow coordination of huge workloads across horizontal clusters of computing resources. New levels of speed and scalability are possible thanks to the cloud, including the ability to surface hidden insights in raw data. And they’re just as necessary, given the enormous increases in data of all types that needs analysis and visualization.
Question 3: Do we have data quality issues that undermine user trust?
Now that you’ve found your data and moved it to where it needs to go for better analytics, doubts may begin to creep in. “Can I actually rely on this data? Is it usable? Is it clean? Are there quality issues I can’t ignore any longer?” This last question is integral to building a strong data culture. It also begins to beg questions about data protection in the cloud, such as: “How can I be sure my sensitive assets aren’t getting into the wrong hands or being used inappropriately?”
Solution: Data Quality and Governance
Data Quality and Data Governance go hand-in-hand and represent the last mile of a data management journey because they touch directly on goals like turning data into a credible, trusted view of each customer, supplier, and product and delivering trusted data for trusted business insights.
Though, here technology is not the complete answer. A company can have the best technology in the world, but without the people to implement change management in the organization, it's unlikely to be adopted. That’s why much of our focus is to deeply understand the personas who touch the data and get at what they need from a common metadata foundation or a common governance foundation.
In fact, rather than making this strictly a data governance question, we like the concept of data empowerment as a holistic approach to managing data that spans the governance teams and all data stakeholders, as well as the policies and rules they create, and the metrics they measure success by. Data empowerment is how you get the right data to the right users at the right time, while maintaining consumer trust and ensuring compliance with both external regulatory mandates and internal privacy policies.
It’s on this foundation of data empowerment that you can deliver the right data to the right consumers with the right quality and the right degree of trust and drive business outcomes. Once you answer the first three questions, of course.