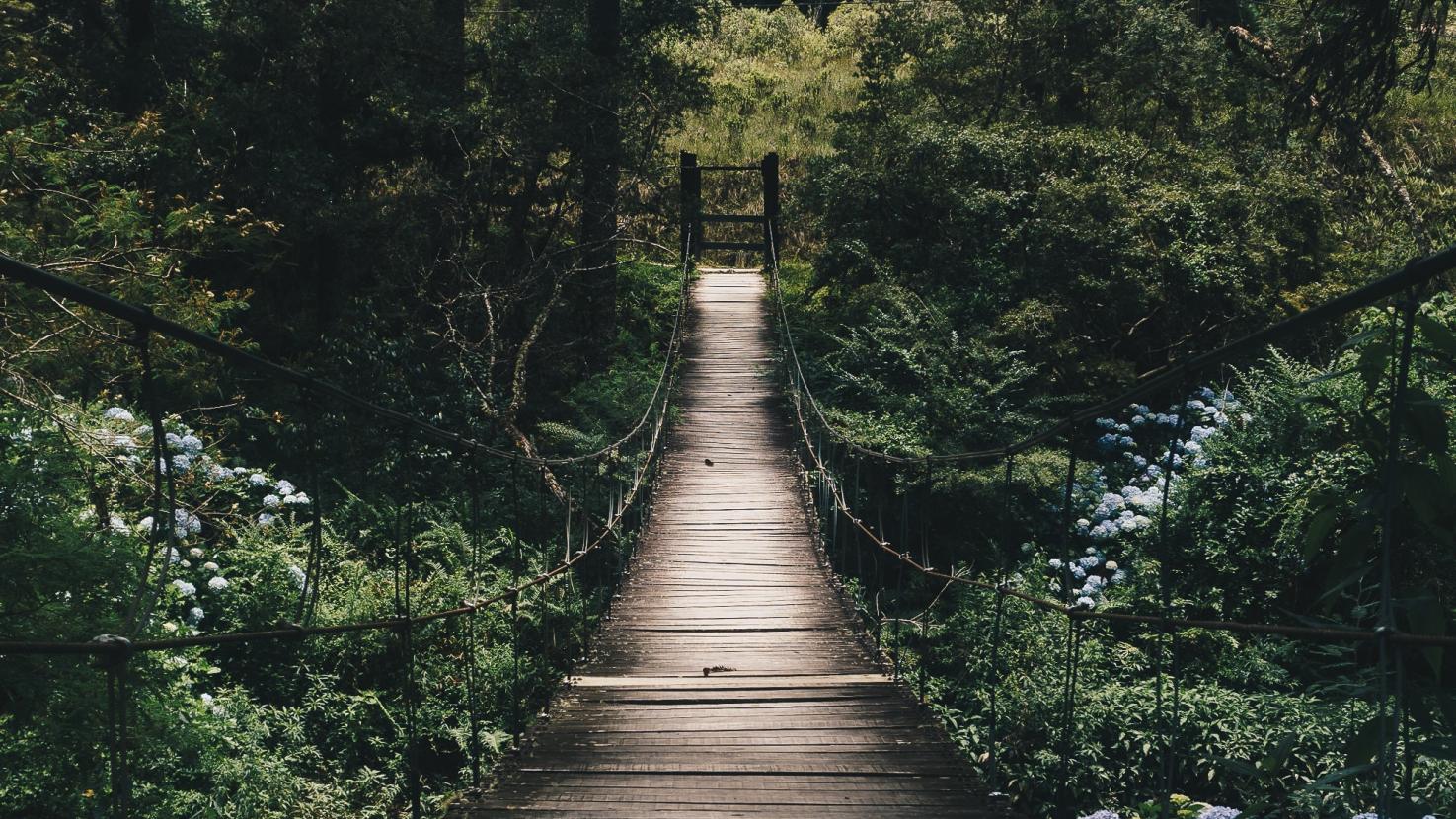

Data Governance vs. Data Management?
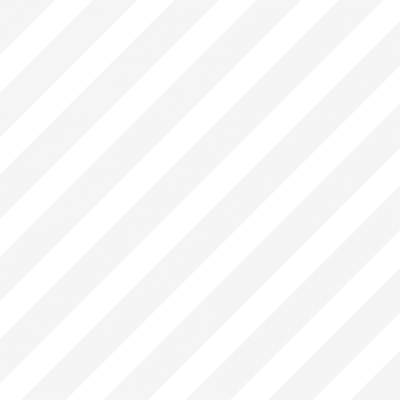
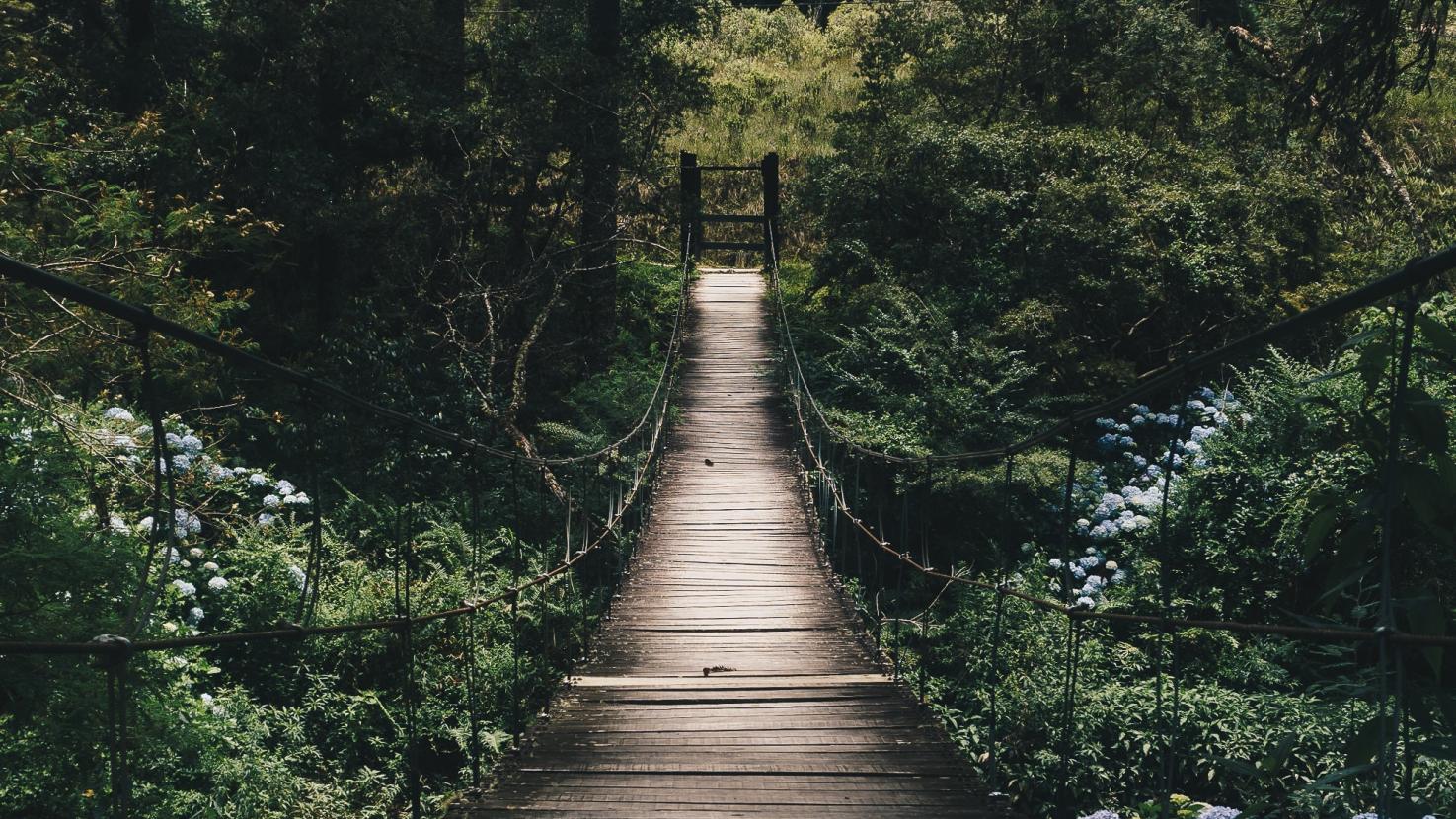
Organizations that excel at delivering insights through data all have one thing in common: clear boundaries. They know who does what, particularly in regard to data governance vs. data management. They also know what provides value to the enterprise. It’s easy to assume that every other company does a better job than you, especially when those fancy brochures claim enormous value from data, or you see a speaker at a conference talk about how everything turned around when they joined an organization. But not everyone is doing better than you, and most don’t win awards.
Clear scope required
One of the things I’ve been advocating for a long time is a clear scope for data governance. Certainly, it would be nice if the industry could agree on what that is, but I’ll take your organization agreeing as a win. Just like an onion – or perhaps a cake is a better-tasting analogy – there are layers to the clarity and scope conversation, and in this article I want to dive into the differences between data governance and data management. Both seem to be the ill-defined, not-fun part of delivering data insights. As a result, neither gets a lot of love. It is so much more fun to talk about how to visualize data or create an educational program that increases the literacy or fluency of your workforce, but none of that matters if you can’t appropriately manage the data along the lifecycle.
Where data governance lives
Two scenarios cover most situations for data governance. Where data governance lives is important because differences in scope and accountability spring from the roots of your organizational structure.
The first and most common arrangement is that data governance is buried somewhere in an IT function. If this is the case, your ability to work side by side with the business to create contextually driven definitions of your key business terms is the biggest lift. Once you complete that effort, collaborating with your peers in data management to deploy those terms into the data repository is a shorter walk.
The second arrangement, less common but preferable, is that data governance sits in the business. Why is this preferable? Because the primary function of data governance is to create a bridge between the data and the insight. It’s hard to do that without context, and the best way to build context is to understand deeply what your business does. If you’re in data governance and embedded in the business, you can easily drive those context-driven conversations. However, partnering with data management to deploy terms or set data quality standards can sometimes feel like you’re taking one step forward and two steps back when the two teams aren’t well aligned.
What do data management and governance have to do with each other?
Everything. The act of governing the data gets so much attention that people forget the whole point. What is the point, you ask? Well, it really comes down to consistency. Yes, I talk a lot about the four operational pillars of governance and consistency is not among them, but the reality is that the foundation provided by these pillars creates a powerful consistency you can leverage to increase usage, quality, lineage, and protection. You can’t gain consistency if you do not deploy the work into the data repository*, hence the importance of data management.
The overlap between data management and data governance is significant. Not just because a lot of the work lies in the intersection of the two, but because we use the same or similar terms for distinct work efforts. For example, both data management and data governance are responsible for metadata but the purpose of each is different. Both use concepts of data modeling (or should) but that last mile – either creating physical tables or defining context and relationships – is different. Data engineering is the primary domain of data management, but most data governance professionals will tell you that data engineers are their go-to resource when work is being done in the data repository. The overlap creates complexity especially when there is a lack of clarity.
To realize the value of data governance, you must change everything from shared objects in a semantic layer for use in dashboards to data quality parameters. How that gets done is often at issue. Whether it’s managed as distinct projects or encompassed as part of the team’s work, the alignment between the data management versus the data governance teams are often at odds. It’s not uncommon to have a data governance team work hard to define a term and create parameters and build a lot of good will with a stakeholder, only to have that work stalled in deployment because you didn’t have alignment with the data management team. The murkiness about the roles gets in our way.
I have developed this short list to illustrate some of the potential overlaps between data management and data governance.
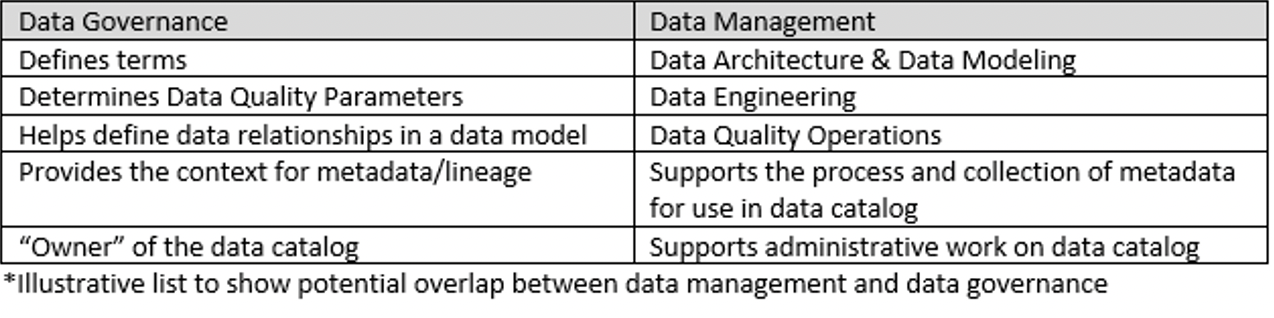
Who Does What? And why? For how long? When?
When my son was small, he watched an animated series called “Busy Town Mysteries.” It ran exactly as it sounds. In a busy small town there were always mysteries to solve. The rising action of the story arc typically started when someone would lose something (an all-too-common occurrence when you’re the parent of a small child). The main character would sing a ditty that included the line, “Who, what, when, where, why and how much?” Those are the questions we must ask ourselves when we’re working on solving the mystery of how data management and data governance work together.
Here's an exercise I encourage you to do. Sit down with your data governance team and your data management team and write some guiding principles. This document should help you navigate how to work together going forward. It should be aspirational, certainly, but more importantly it should be operational. In other words, you can refer to it to know who does what when. The aspirational part should be the “why” (which without a doubt should be inclusive of the organizational strategy and increasing usage of the data). From these guiding principles the manager or leaders of the teams can sit down and decide what those handoffs look like. It’s not the fun stuff for sure, but knowing exactly what happens when and by whom is an empowering place to be.
*I use the term “data repository” as a general term to refer to anything that remotely looks like a data warehouse, data lake, data lakehouse, data cloud, data McMansion, you get the drift.