
Twelve Barriers Inhibiting the Progress of Data Literacy and Twelve Solutions to Accelerate Growth
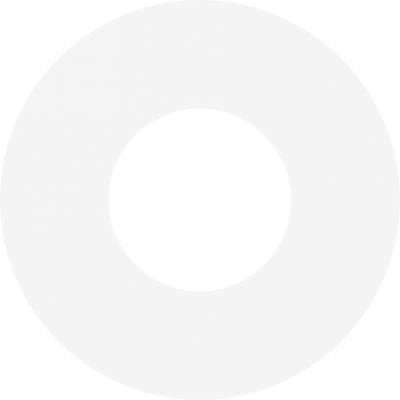
Data Literacy is about much more than knowing how to create fancy charts. It’s about looking at one’s environment through the lens of data. It’s about applying what is gleaned, often in a team setting, in order to achieve goals and objectives.
An organization’s ability to drive data literacy hinges on the attitudes and behaviors of its leaders. A leader can set the stage for collaboration that unlocks the value of data, or they can drop the curtain on the whole affair, putting an end to any progress their team might have been making in this critical area. Which role leaders decide to play, and how much time and energy they devote to that role, will make or break any organization’s data literacy initiatives.
The fact that I view the leader’s role this way is the reason I was interested in engaging with other leaders in two braindates hosted by Tableau’s brand new community – The Data Leadership Collaborative – in May of 2021. Over the course of two hour-long sessions, I was able to interact with nine different leaders – six women and three men – from the U.S., the UK, and Australia to identify both barriers and solutions to organizational data literacy.
The group had an active discussion around the top top barriers inhibiting progress on data literacy:
- Lack of Ownership: Who owns data literacy within an organization? If no one owns it, there is an increased risk that no progress will be made.
- Executive Partnership: Do executives know how to best interact with data teams? Sometimes they’re simply looking for ad hoc reports or insights, but these can sometimes amount to unreasonable requests
- Challenges with Charts: People might not feel comfortable reading & interpreting charts: “What does this bar chart tell me?” Do team members need an analyst to sit down with them and tell them what it means, or do they have the confidence to make sense of it themselves? Are the charts themselves poorly designed or constructed, such that they’re more confusing than elucidating?
- Trouble with Tools: Can our people do more than use tool X out of the box. Or is this the wrong focus?
- Data Communication Skills: Do our team members have the presentation and communication skills to effectively convey the most important and relevant insights in our data, or the full story if there is one? Or are people instead getting stuck in the weeds, and missing the opportunity to get the critical message across?
- Dizzying Disparate Data: Are we able to bring together data that might be scattered amongst different systems and locations? Can we prepare that data for analysis? This can be a huge barrier that stops effective data analysis before it even starts
- Hoarding Data: There are necessary and appropriate limitations to put on the access to data for privacy and security reasons, but are people preventing others from accessing data for the “wrong” reasons, such as power plays or agendas? Doing this can be toxic and will certainly hinder data literacy.
- Cultural Blockers: Cultural factors can prevent us from achieving “data ubiquity.” Executives might have wrong expectations, good guides might be lacking, communication might be stilted or ineffective, data might feel intimidating and complex, and anything but simple, lingo / terminology might be overused and poorly understood
- People are Asking, ‘So What’? Is the end goal of data literacy just a bunch of dashboards? Or is it more than that? If all the organization is doing is creating fancy dashboards that no one is using, then the organization is missing the chance to actually use data. Instead, they should look at the workflow of how decisions are made, how tasks are carried out, and facilitating decisions with speed.
- Missing the Target Audience: Who are the audience members, and what do they need to do with the data? How can training be customized to their unique needs & situations? If these questions aren’t asked and answered, then the data tools, presentations, and training will miss the mark.
- One-Size-Fits-All: Data and training can’t involve a one-size-fits-all approach. Training by role, persona, or track as opposed to one-size-fits-all: execs vs front line workers. Data consumers vs data workers. These individuals need very different training and resources.
- Not Measuring Progress: How well are we doing? Are we moving in the right direction/ If we don’t blend both subjective vs objective measures of success, we won’t know if we’re achieving our data literacy goals, or even what those goals are.
But of course, we couldn’t stop at just identifying the barriers! Collectively we brainstormed some creative solutions that we thought could help accelerate everyone’s efforts.
- FIRST! Ensure Data Governance, Integrity, Ethics: Do you really want a team that’s highly data literate if they aren’t committed to doing good rather than doing harm with data?
- Accentuate the Positive: Promote positive uses of data - the “Data Hero” - to help people recognize what good behaviors look like. Socialize success stories
- Get the Branding Right: Is “Data Literacy” a good / useful term, or are there any issues with that term? Some might feel insulted by the notion of learning to become “literate”. Find the right term for your group
- Top Down & Bottom Up: Ensure that you have both executive sponsorship and also grass-roots support, otherwise still resistance will arise.
- Appoint an Overall Data Literacy Owner: Find someone with top leadership support and trust to own the overall culture change initiative.
- Identify Internal Champions: Identify multiple “Data Champions” or “Data Cheerleaders” on each team to be the go-to person that anyone on the team can approach ask questions
- Make it Relevant: Find the “burning questions” and show how they can be answered with data. This approach ensures the content is immediately relevant to the people who are on the learning journey.
- Make Data Accessible: People don’t always know what data is available to them, how to access it, or what it even means. Making data visible and giving people the vision of how it applies to them is a critical part of unlocking data literacy within an organization.
- Set Realistic Expectations: Data isn’t a panacea, and it won’t necessarily solve all of their problems. Questions will arise, disagreements will surface, and what the data is telling us isn’t always clear.
- Get the Order Right: Don’t try to invest in expensive technology if knowledge and skills are lagging so far behind that the deployment will fail.
- Make it Okay to Have “Beginner” Questions: If people are afraid of raising their hand and asking a basic question, or cultures where people who don’t know something are ridiculed, are cultures
- Define the Levels of Capability: What are the distinct levels of knowledge & skill at the company, and how can we help people identify their current “Data Literacy Level” and find ways to progress to the next level?
I found it amazing and refreshing that this relatively small group of data leaders from around the world was able to identify so many common problems and solutions in such a short amount of time. I’d like to say thank you to Candra, Sarah, Michelle, Pam, Cesar, Rob, Wendy, Cindy, and Paul for their active and enthusiastic participation.
It’s a testament to their perspective and experience, and their willingness to share it with others. This knowledge-sharing and the associated human connection is a hallmark of a thriving data community. I’ll be scheduling more braindates in the near future. Feel free to contact me if there are topics you’d like to discuss together.