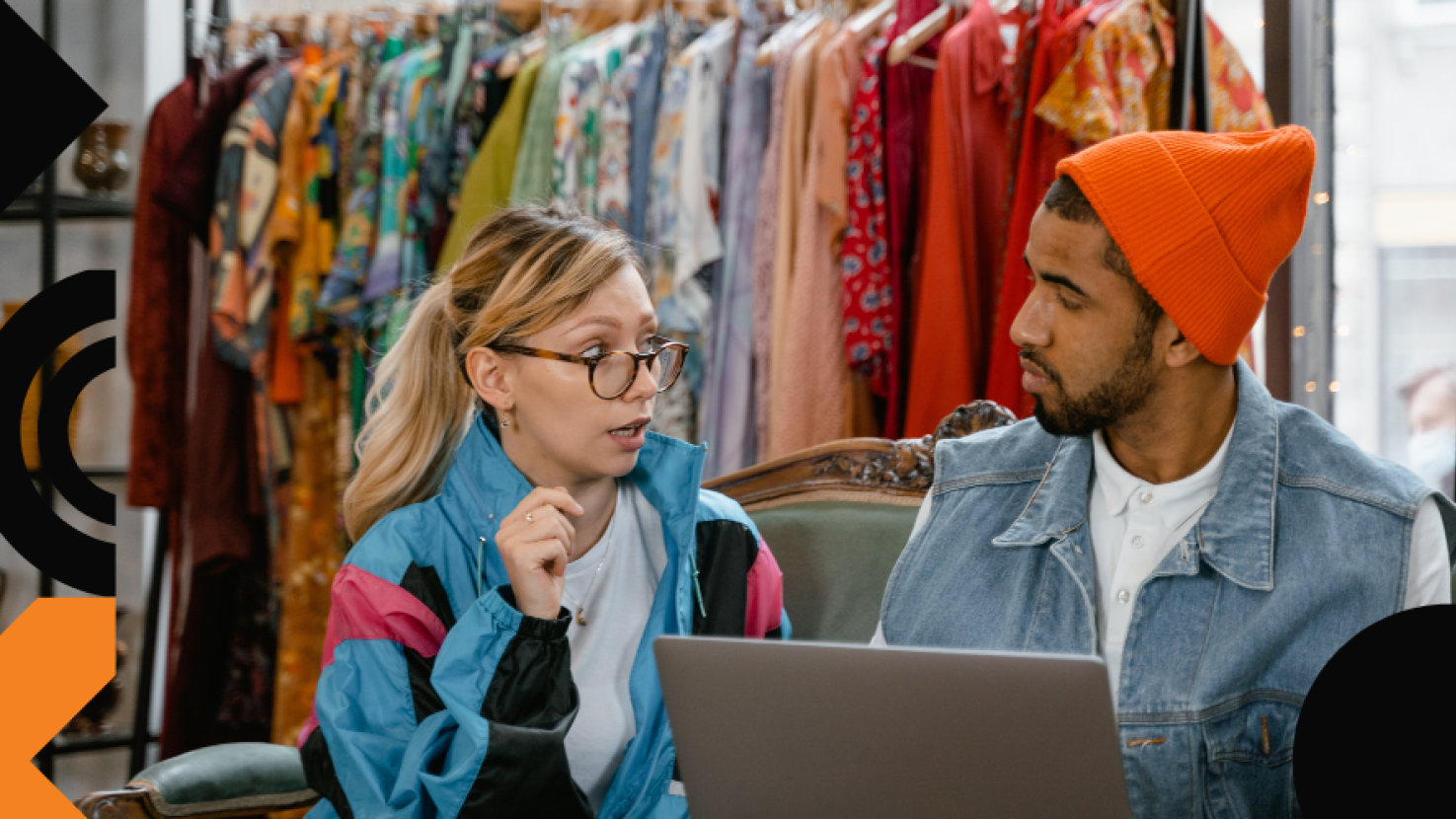

Why predictive analytics is the future of the retail economy
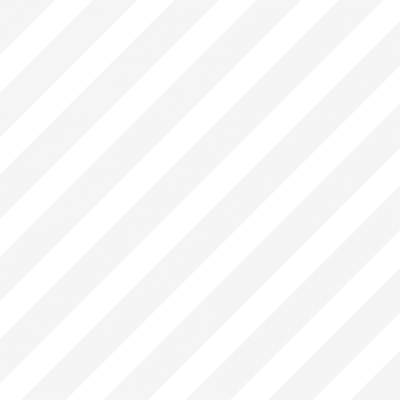
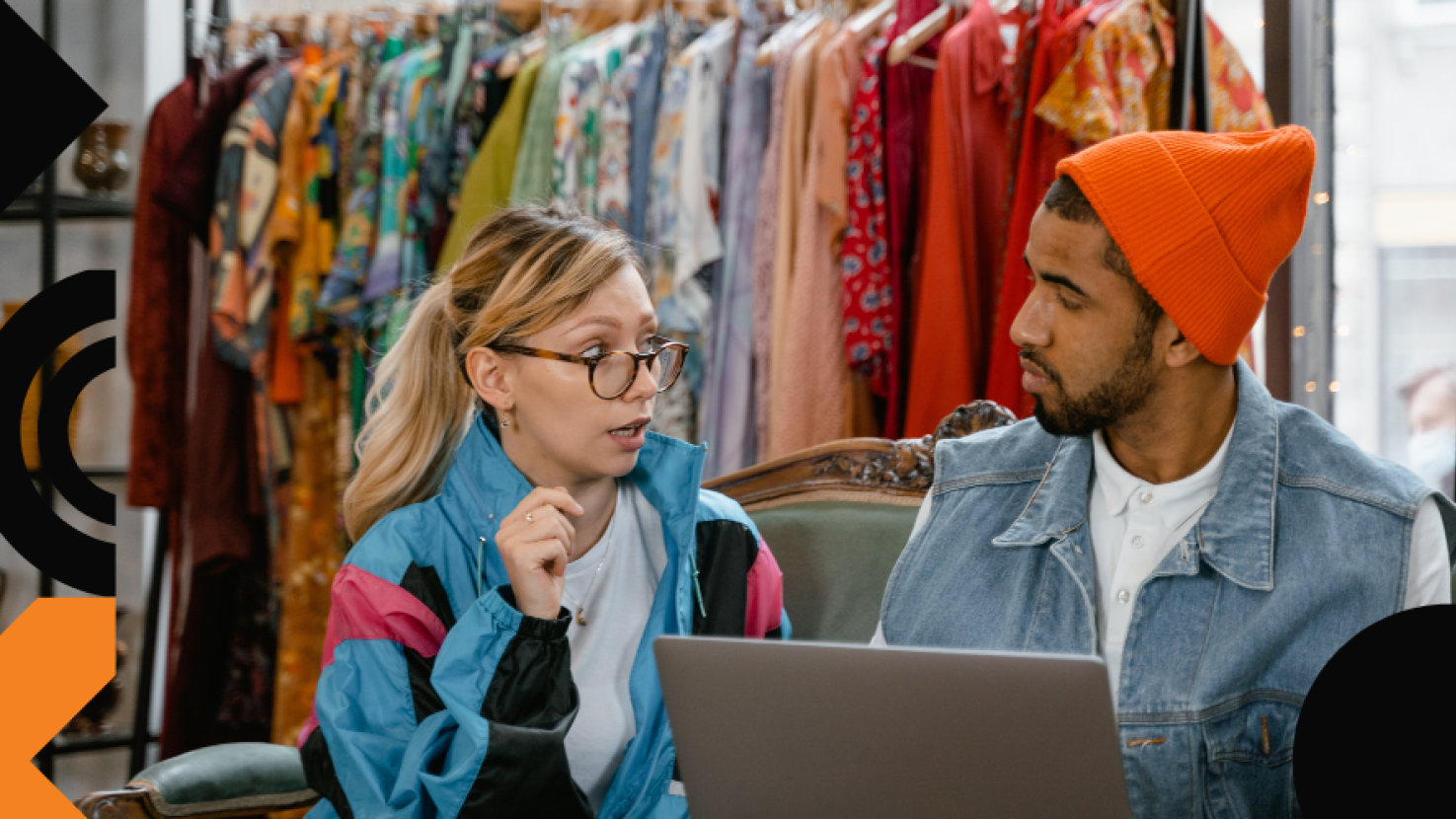
With a forecasted annual growth rate of nearly 30 percent, data analytics is becoming more pervasive all the time. At the front of the analytics pack, of course, is predictive analytics. What company out there wouldn’t want to have a more accurate view into its future outcomes or the likely results of its strategies? A few dynamics make this the most opportune time ever for predictive analytics.
First, data is more accessible. Technology constraints in the past – including the high cost of storage – meant that collecting and preserving data was once a lot more time consuming and expensive. These constraints are lessening.
Second, data is more plentiful. Whether it is coming at you through social media, customer interactions, marketing campaigns, IoT devices, or smart devices, there is no shortage of channels through which you can receive a steady stream of high-quality data.
Third, data integration tools are more powerful. Data transformation and cleansing tools can create single-source-of-truth data for each customer. Once data is integrated in this way, it is much easier to make sense of for downstream use.
The missing link: aligning analytics to business objectives
I would argue that for all this progress, many organizations don’t have a clear strategy of business outcomes and objectives they want to achieve with data analytics. You can have a data set many times larger than your competitors sitting in your data lake, but if you don’t have a way to make sense of it and use it to achieve the right outcomes, all you have is a collection of siloed data.
Retail is an excellent example. Predictive analytics is key in such a fluid industry. If I want to launch a new food product, how do I know that it will sell well in specific regions? Here in the UK the north and south differ in many ways. Southern England has a younger population based on the cultural and employment opportunities London provides, but as you go north the population becomes more diverse. With retail predictive analytics you can make informed choices based on many scenarios:
- What would shoppers do if we launched a new product while discontinuing another one?
- What would happen if I created more choice by adding an imported product to one where a domestic provider may be faltering?
- How would competitive pricing differ for products based on ethical sourcing, and what is the upper limit consumers would be willing to pay?
With predictive analytics in retail, it’s possible to conduct many analyses using the historical data based on buying patterns and shopping behavior. For example, with your own customers you could create a predictive strategy to understand:
- how your competition is using data in their omnichannel strategies
- how seasonal uptake may influence your supply chain needs
- how shifting demographics may impact sales for different products
- which of your channels are performing well, and which are shrinking
- how different sales strategies might increase basket size
- how you can use real-time inventory and stock updates to make your supply chain more resilient
Getting the right start on predictive analytics
If you are a data leader and find yourself promoted to a more senior decision-making role, what can you do to create momentum around predictive analytics?
Here are five strategies I’d suggest:
- Honestly assess the maturity and adoption of data strategy in your organization. Does a robust data strategy even exist? If there is one, where does the team stand from a maturity perspective? Do they have a track record of success in predictive modeling? If that is the case, how can you ensure continuous innovation? If maturity isn’t high, what are the key enablers you should put in place to take them through the journey?
- Align data to business outcomes. The biggest challenge any data leader faces is organizational culture. Everyone, wherever they stand in the corporate ladder, needs to understand the value of data. And that data value realization must be persona-based. To deliver on the vision, you need to have cross-functional, high-performing teams who constantly look into models and the data so you can make sense of it.
- Take a data life cycle view. Too often, data still sits in disconnected silos and different formats. In addition to collecting data, you must enforce a practice of cleansing, maintaining it, and harmonizing it to turn it into actionable insights. Although healthcare has defined industry standard templates to exchange data, groups such as GS1, the National Retail Foundation, and ISO continue to work on standards for consistent retail data exchange.
- Build toward key success factors. By building predictive modeling, machine learning, and data mining tools, we can extract insights from collected data and use it for applications like future trend analysis and retail product demand forecasting. Creating a vision of what these tools can help your organization to attain in becoming more customer-centric makes them a much easier sell at the senior executive level.
- Measure outcomes and revisit goals. With consistent data practices, you can define KPIs to measure impact and revisit your outcomes regularly. If your strategy is not paying off, or if you want to change direction, you can realign based on results.
Of course, whether you’re doing predictive analytics in retail or any other industry, the final step to make predictive analytics successful is to evangelize. As a data leader, you must lead the charge to collect data from customers at every interaction with the business, identify the right technology stack to meet your goals, and create a culture of data-driven decision-making. In today’s retail landscape, it is data and its intelligent use that truly separates the winners from the losers.