
Four Ways to Win Hearts and Minds During a Data Transformation Journey
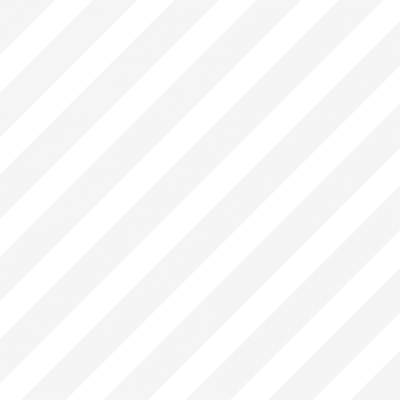
In my data and analytics organization, we have been on a transformation journey for nearly three years, bringing disparate analytical teams together into one large organization of about 580 people. As we’ve assembled this team we’ve also been moving away from individual tools such as Excel or saving things on desktops to more modern practices and platforms.
One of the things we have wrestled with more broadly is that when you deploy a solution that radically disrupts the way your organization works, it disrupts on several fronts:
- It disrupts people, because you find you don’t need all the staff that you currently have
- It disrupts training, because it requires new skill sets that only a handful of team members may have
- It potentially disrupts culture, especially if the solution has existing associations based on its use by entities like investigative agencies (many types of firms rely on crunching data, but rarely with the same end in mind)
Considering that these serial disruptions are not unique to the NHS, winning hearts and minds during a data transformation journey is something every data leader will face in their career as well.
Four strategies for success
What should data leaders be planning for? Here are four recommendations based on my experiences over the past few years:
- Be open and empathic with your staff. This will include working with those staff who resist change, those on the fence who can be brought along, and what I call the futurists, who can become champions of new technology going forward. Whatever you do, avoid creating a smoke and mirrors environment where you introduce a technology as a stealth effort. Be honest and say, “This technology is coming, and this is what we expect it to do.” It’s too easy to hire a strategy consulting house and say, “Here’s the operating model” without engaging your staff. But just because staff engagement about new technologies can be painful and difficult doesn’t mean you can skip it.
- Have strategies at hand for working with each category of team member. Change-resisters, for example, may benefit from retraining or be open to conversations about their exit path. Fence-straddlers tend to recognize that their current ways of working fall short, and can be brought along with training on the parts of the new technology that address their pain points. Finally, futurists should be identified and brought on board quickly, because they often represent the future of your organization.
- Work with software vendors as collaborative partners. Traditionally a company relies on a software vendor to sort everything out for them initially. I believe that vendors need to work more in partnership with clients post-go live, which includes helping to develop internal champions so their software has a higher chance of success.
- Continuously look at acquired technologies and update them as needed. Whatever we choose as a data and analytics solution today is not necessarily the solution that will last for five or ten years, so we as senior leaders need to be open to the idea of being challenged by users who are evaluating what we've acquired. Does it work? Does it not work? Can it be improved? I like the concept of having a Red Team, whose role is to look at our existing solutions and technologies on a daily basis, try to determine what isn’t working about them, and report back to senior management. This is a very successful practice in cybersecurity, so why not use it in data and analytics?
Four lessons learned
No one embarks on a data transformation journey without learning a few lessons. In our department’s case, here are a few:
- We should have engaged with our team members up front about what the future could like. As a result, a lot of our progress slowed considerably at the six-month mark. That meant we had to rapidly pivot and develop the idea of champions. As we empowered more of our senior leaders to take roles in the change management process, progress rapidly picked up again.
- We could have been more aware of the mixed maturity of our team. On the one hand, we had staff who were fully buying into the vision and wanted to move forward quickly. They needed R, Python, a new laptop, and access to data. They needed to plug into our new operating model, which is a federated matrix structure where everyone can work on a variety of projects. We probably could have moved faster with this group. On the other hand, we could have done a better job with the fence-straddlers who were slower on the journey. Allowing them to exist in both worlds and creating a mixed economy of projects turned out to be a good transition plan.
- We needed to invert our leadership pyramid. When we looked at some of the champions we needed for new disciplines such as Python and data engineering, these people were not our director-level recruits. They were junior staff, some from startups, who had fluency in Python and benefitted from our help building their teams. But to make the overall organization work, many of us needed to absolve ourselves from senior-level responsibility and actually hand it down to form communities of practice. This is easier said than done at first!
- We needed to put the right people on the management track. Another part of winning hearts and minds is recognizing that even some of your best practitioners can pass the baton to others and become more managerially focused. Realize that these colleagues may have grown teams and stood the test of time and change as coders. They probably know the ins and outs of your culture. And you can play a vital role in helping them pivot to sharing their data and analytic skills with others, because it’s not all about crunching numbers. Management in data science is an art one that’s often forgotten. This does not, of course, mean dropping them into a new role and walking away. You must equip them with tools and knowledge, give them a bit of runway, and provide them with peer support from fellow managers as well.
In my own case, I’m still a clinician at the end of the day, but I’m now a manager as well. I’ve always had the support of my senior leaders and peers on my management journey. They’ve mentored me in the things managers face, like dealing with difficult staff members, creating product teams, and starting to work in an agile way. You’re rarely taught these skills as an analyst.
As you move from legacy technology tools to new ones and downshift from existing skill sets to more modern practices, don’t forget the people behind the titles. By following business change management practices with empathy and an open mind, you can help disruption to feel more like positive evolution.