
From Descriptive to Prescriptive: How to Create Data Impact
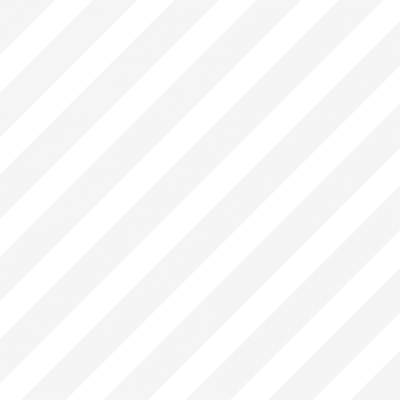
Any organization with its sights set on data leadership should place a premium on the ability to describe what happened in its past, know to a degree of certainty why it happened, predict what could happen next, and prescribe what actions are needed to continue to flourish.
Of course, I’m speaking of the data and analytics capabilities that carry an organization from hindsight to insight to foresight – descriptive, diagnostic, predictive, and prescriptive – all of them ideally built on solid data engineering and management to ensure that the data is both available and trustworthy.
Rather than focusing on one area, the Commercial Data and Analytics organization in my last role at illumina focused across the range of all four. Here are some of the questions we set out to answer in each area and the tools we used in our work to support the success of our business.
Descriptive Analytics: Descriptive analytics is about delivering the right information to the right people at the right time in order to drive action. From a sales standpoint, we were looking into how trending had happened in the past, but also the growth rates across different customer bases, market segments, and platforms we sold.
- Tools We Developed: We produced balanced scorecards that aligned key performance indicators across the organization as well as weekly insights push reports that delivered relevant information on metrics like shipments on a consistent basis.
Diagnostic Analytics: In this area we double-clicked into our data to understand what really happened during a quarter or how the pandemic was having an impact on our business. We did the same thing on the services side of our business, providing insights to our field technicians so they could optimize where they spent time.
- Tools We Developed: We created business trends dashboards that could answer specific business questions and align to existing business process workflows, as well as customer insights dashboards that combined multiple data sets into a single, holistic view of customer activity. Trending data here helped us be more anticipatory than reactive with our customers, which built their loyalty.
Predictive Analytics: Here we provided data science support for our instruments using telemetry data to predict when something might go down. Despite having tens of thousands of instruments deployed in the field, my team provided alerts out to the user base to say, for example, “Hey, we have an issue with your chiller.” Even better, we could reach out to say, “There’s an issue with this sequencing run you’re seeing, so if you take this preventative action you can save it.” This was highly appreciated in settings where a single sequencing run can cost tens of thousands of dollars.
- Tools We Developed: Our proactive instrument alerts helped us predict and service the needs of customers faster and allowed us to resolve cases 25% faster when customers were connected via telemetry. And our forecasting predictions brought together multiple data sets to boost predictive power using a machine learning approach to supplement human intelligence. This is part of what we called our illumina Proactive Program.
Prescriptive Analytics: Finally, our goal with prescriptive analytics is to make data-driven recommendations for business leaders to consider for action. These recommendations are based on how customers are actually engaging with us, not how we think they might be.
- Tools We Developed: We delivered business process optimization insights that were aligned to our existing business process to create “Digital Twins” for what-if scenario planning. We also did customer clustering analysis to identify customer profiles through real-world buying patterns.
A key element of these initiatives is that we integrated the intelligence we generated into our applications and workflows, so not only into Tableau, for example, but into Salesforce as well. Whenever possible, we wanted to minimize people running around looking for data when they shouldn’t have to.
Ultimately, I think our data efforts were successful because they did two things: they empowered people with information, and they also used data to make them accountable.
You can’t achieve one without the other, but when you do both the impact is tremendous.